Unlike existing robots on the market, such as Boston Dynamics’ Spot, which moves around using internal maps, this robot uses cameras alone to guide its movements in the wild, says Ashish Kumar, a graduate student at UC Berkeley, who is one of the authors of a paper describing the work; it’s due to be presented at the Conference on Robot Learning next month. Other attempts to use cues from cameras to guide robot movement have been limited to flat terrain, but they managed to get their robot to walk up stairs, climb on stones, and hop over gaps.
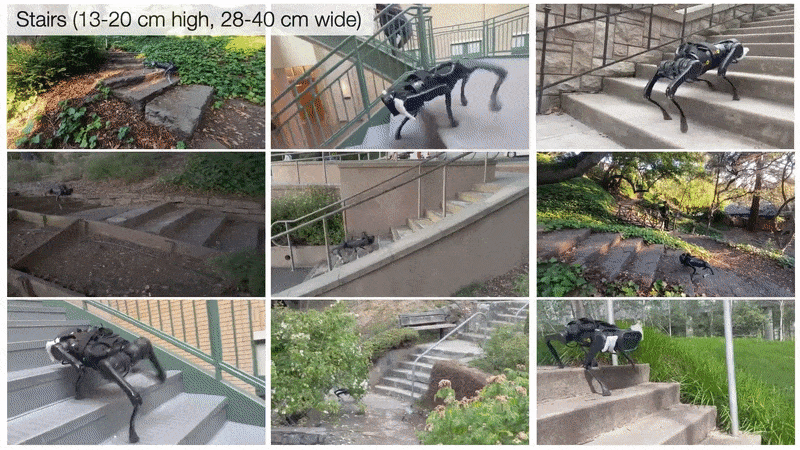
COURTESY OF THE RESEARCHERS
The four-legged robot is first trained to move around different environments in a simulator, so it has a general idea of what walking in a park or up and down stairs is like. When it’s deployed in the real world, visuals from a single camera in the front of the robot guide its movement. The robot learns to adjust its gait to navigate things like stairs and uneven ground using reinforcement learning, an AI technique that allows systems to improve through trial and error.
Removing the need for an internal map makes the robot more robust, because it is no longer constrained by potential errors in a map, says Deepak Pathak, an associate professor at Carnegie Mellon, who was part of the team.
It is extremely difficult for a robot to translate raw pixels from a camera into the kind of precise and balanced movement needed to navigate its surroundings, says Jie Tan, a research scientist at Google, who was not involved in the study. He says the work is the first time he’s seen a small and low-cost robot demonstrate such impressive mobility.
The team has achieved a “breakthrough in robot learning and autonomy,” says Guanya Shi, a researcher at the University of Washington who studies machine learning and robotic control, who also was not involved in the research.
Akshara Rai, a research scientist at Facebook AI Research who works on machine learning and robotics, and was not involved in this work, agrees.
“This work is a promising step toward building such perceptive legged robots and deploying them in the wild,” says Rai.
However, while the team’s work is helpful for improving how the robot walks, it won’t help the robot work out where to go in advance, Rai says. “Navigation is important for deploying robots in the real world,” she says.
More work is needed before the robot dog will be able to prance around parks or fetch things in the house. While the robot may understand depth through its front camera, it cannot cope with situations such as slippery ground or tall grass, Tan says; it could step into puddles or get stuck in mud.