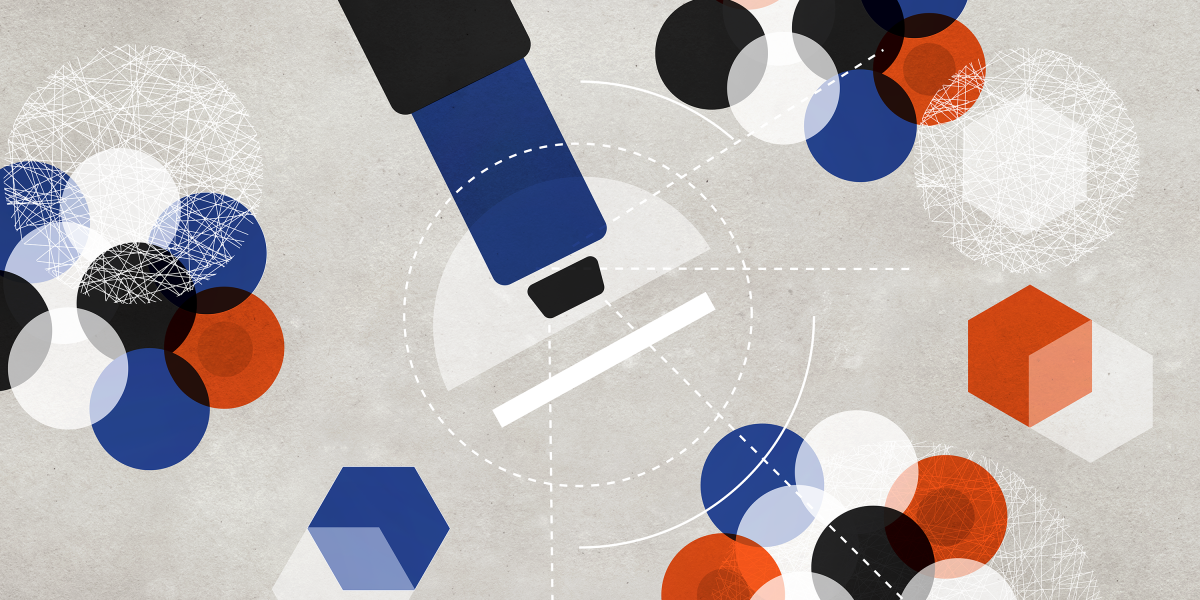
It’s been exciting to see technology that rewrites and improves what we thought was an established health concept—how to evaluate the need for knee surgery, for instance. With the help of technology, we can focus resources on areas of human health that are complex and chronically understudied, or we can move on from simply naming inequity issues to fixing those issues. If AI models can highlight places where our society is failing people, those people could have better options. It’s also heartening to see a new focus on reproducibility and benchmarks in AI research.
What ML and AI in biotech broadly need to engage with are the holes that are unique to the study of health. Success stories like neural nets that learned to identify dogs in images were built with the help of high-quality image labeling that people were in a good position to provide. Even attempts to generate or translate human language are easily verified and audited by experts who speak a particular language.
Instead, much of biology, health, and medicine is very much in the stage of fundamental discovery. How do neurodegenerative diseases work? What environmental factors really matter? What role does nutrition play in overall human health? We don’t know yet. In health and biotech, machine learning is taking on a different, more challenging, task—one that will require less engineering and more science.
Marzyeh Ghassemi is an assistant professor at MIT and a faculty member at the Vector Institute (and a 35 Innovators honoree in 2018).