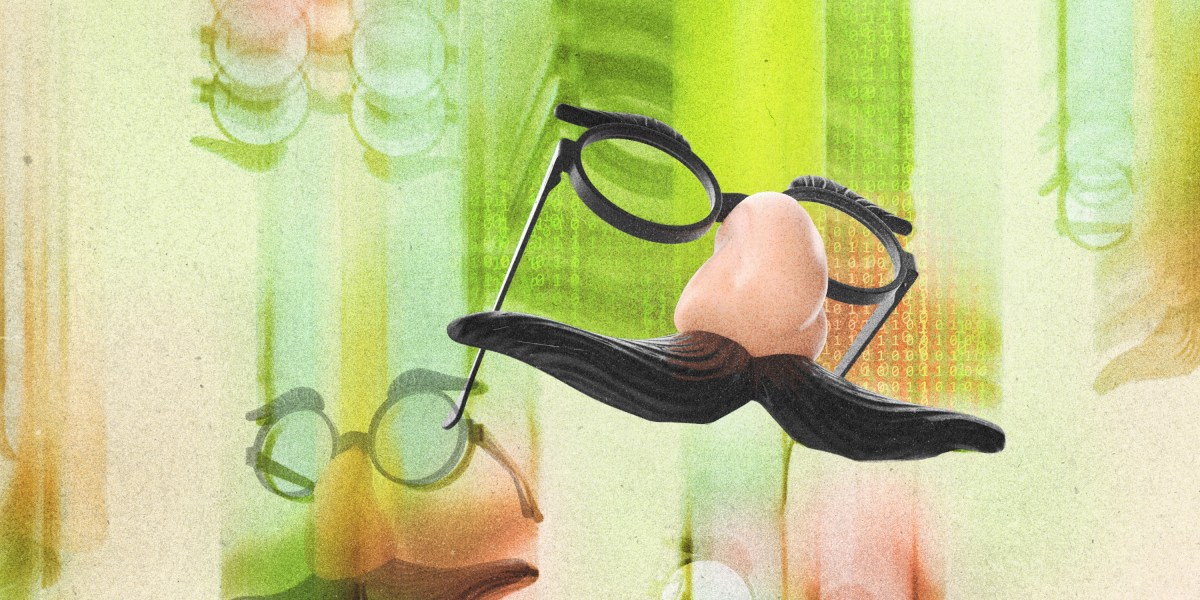
Recently we’ve seen some AI failures on a far bigger scale. In the latest (hilarious) gaffe, Google’s Gemini refused to generate images of white people, especially white men. Instead, users were able to generate images of Black popes and female Nazi soldiers. Google had been trying to get the outputs of its model to be less biased, but this backfired, and the tech company soon found itself in the middle of the US culture wars, with conservative critics and Elon Musk accusing it of having a “woke” bias and not representing history accurately. Google apologized and paused the feature.
In another now-famous incident, Microsoft’s Bing chat told a New York Times reporter to leave his wife. And customer service chatbots keep getting their companies in all sorts of trouble. For example, Air Canada was recently forced to give a customer a refund in compliance with a policy its customer service chatbot had made up. The list goes on.
Tech companies are rushing AI-powered products to launch, despite extensive evidence that they are hard to control and often behave in unpredictable ways. This weird behavior happens because nobody knows exactly how—or why—deep learning, the fundamental technology behind today’s AI boom, works. It’s one of the biggest puzzles in AI. My colleague Will Douglas Heaven just published a piece where he dives into it.
The biggest mystery is how large language models such as Gemini and OpenAI’s GPT-4 can learn to do something they were not taught to do. You can train a language model on math problems in English and then show it French literature, and from that, it can learn to solve math problems in French. These abilities fly in the face of classical statistics, which provide our best set of explanations for how predictive models should behave, Will writes. Read more here.
It’s easy to mistake perceptions stemming from our ignorance for magic. Even the name of the technology, artificial intelligence, is tragically misleading. Language models appear smart because they generate humanlike prose by predicting the next word in a sentence. The technology is not truly intelligent, and calling it that subtly shifts our expectations so we treat the technology as more capable than it really is.
Don’t fall into the tech sector’s marketing trap by believing that these models are omniscient or factual, or even near ready for the jobs we are expecting them to do. Because of their unpredictability, out-of-control biases, security vulnerabilities, and propensity to make things up, their usefulness is extremely limited. They can help humans brainstorm, and they can entertain us. But, knowing how glitchy and prone to failure these models are, it’s probably not a good idea to trust them with your credit card details, your sensitive information, or any critical use cases.
As the scientists in Will’s piece say, it’s still early days in the field of AI research. According to Boaz Barak, a computer scientist at Harvard University who is currently on secondment to OpenAI’s superalignment team, many people in the field compare it to physics at the beginning of the 20th century, when Einstein came up with the theory of relativity.
The focus of the field today is how the models produce the things they do, but more research is needed into why they do so. Until we gain a better understanding of AI’s insides, expect more weird mistakes and a whole lot of hype that the technology will inevitably fail to live up to.