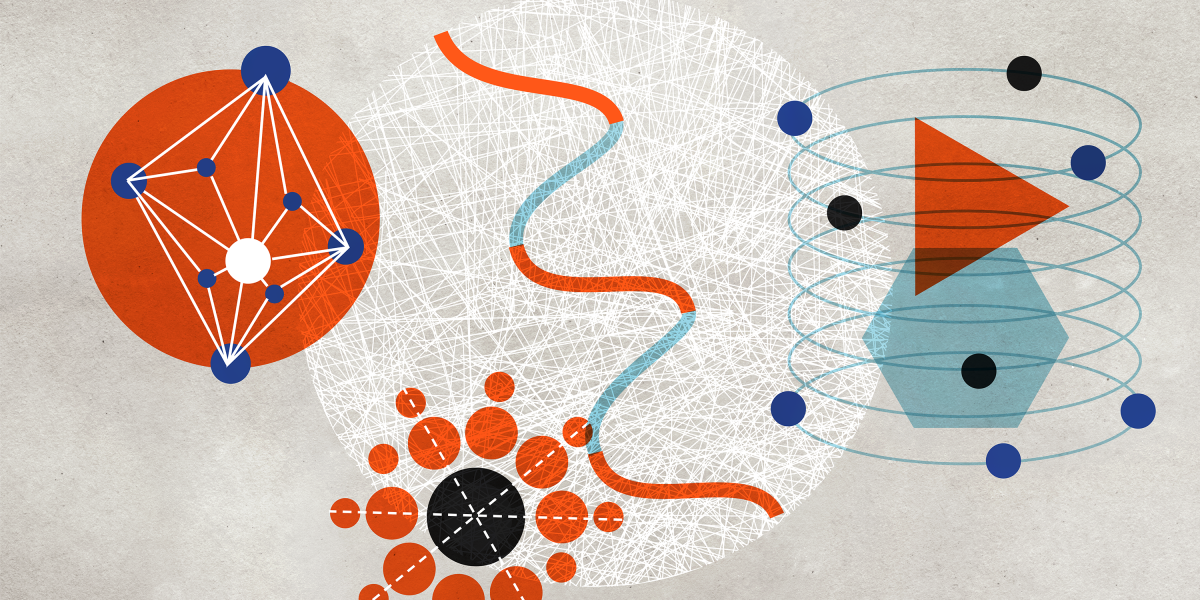
Thanks to this built-in responsiveness, future materials could be made with some decision-making capabilities and adaptability. Intelligent materials may be able to automatically release precise amounts of medication, heal themselves when damaged, or perform logical operations when exposed to light. In fact, some architected materials have already incorporated new kinds of logic gates that respond to either mechanical or chemical stimuli.
One area where I see great potential involves using machine learning to predict new architectures for materials that can emulate computationally trained neural networks using light instead of digital input. Eventually, artificial neural networks could be integrated into architected physical materials to make decisions, eliminating the need to first convert the input into digital signals and then process them in computers. This means materials themselves could someday be made to recognize faces or objects, process language, and classify text or numbers.
To realize this vision, we will need new computational models that can accurately capture the mechanics and physics of the additive manufacturing process for an affordable price. Additional models must be able to perform diagnostics, in real time, to determine whether any defects that form will affect performance.
And as if designing, discovering, and demonstrating new material properties weren’t hard enough, we’ll then have to turn prototypes into technology and manufacture the materials at scale. These tasks represent a major challenge, in part because the models haven’t yet been developed.
Knowing there are many talented people working on these problems, I look forward to the day when we can create architected materials and devices imbued with the ability to make decisions on their own.
Julia R. Greer is a materials scientist at the California Institute of Technology, and was a 35 Innovators honoree in 2008 and a judge for this year’s competition.