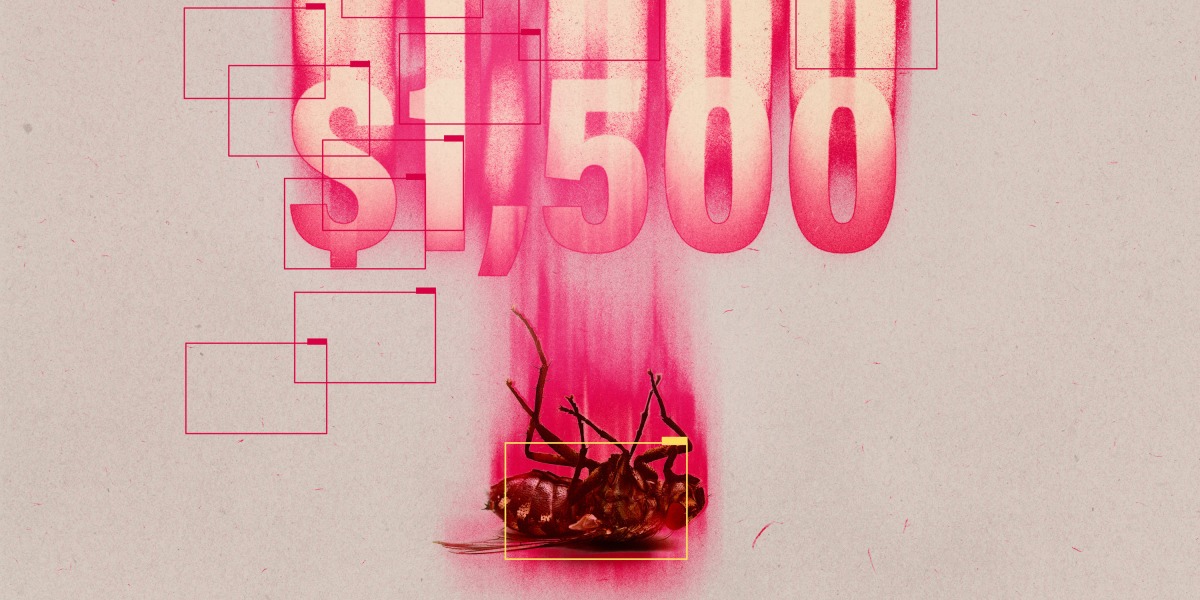
Considering how powerful AI systems are, and the roles they increasingly play in helping to make high-stakes decisions about our lives, homes, and societies, they receive surprisingly little formal scrutiny.
That’s starting to change, thanks to the blossoming field of AI audits. When they work well, these audits allow us to reliably check how well a system is working and figure out how to mitigate any possible bias or harm.
Famously, a 2018 audit of commercial facial recognition systems by AI researchers Joy Buolamwini and Timnit Gebru found that the system didn’t recognize darker-skinned people as well as white people. For dark-skinned women, the error rate was up to 34%. As AI researcher Abeba Birhane points out in a new essay in Nature, the audit “instigated a body of critical work that has exposed the bias, discrimination, and oppressive nature of facial-analysis algorithms.” The hope is that by doing these sorts of audits on different AI systems, we will be better able to root out problems and have a broader conversation about how AI systems are affecting our lives.
Regulators are catching up, and that is partly driving the demand for audits. A new law in New York City will start requiring all AI-powered hiring tools to be audited for bias from January 2024. In the European Union, big tech companies will have to conduct annual audits of their AI systems from 2024, and the upcoming AI Act will require audits of “high-risk” AI systems.
It’s a great ambition, but there are some massive obstacles. There is no common understanding about what an AI audit should look like, and not enough people with the right skills to do them. The few audits that do happen today are mostly ad hoc and vary a lot in quality, Alex Engler, who studies AI governance at the Brookings Institution, told me. One example he gave is from AI hiring company HireVue, which implied in a press release that an external audit found its algorithms have no bias. It turns out that was nonsense—the audit had not actually examined the company’s models and was subject to a nondisclosure agreement, which meant there was no way to verify what it found. It was essentially nothing more than a PR stunt.
One way the AI community is trying to address the lack of auditors is through bias bounty competitions, which work in a similar way to cybersecurity bug bounties—that is, they call on people to create tools to identify and mitigate algorithmic biases in AI models. One such competition was launched just last week, organized by a group of volunteers including Twitter’s ethical AI lead, Rumman Chowdhury. The team behind it hopes it’ll be the first of many.
It’s a neat idea to create incentives for people to learn the skills needed to do audits—and also to start building standards for what audits should look like by showing which methods work best. You can read more about it here.
The growth of these audits suggests that one day we might see cigarette-pack-style warnings that AI systems could harm your health and safety. Other sectors, such as chemicals and food, have regular audits to ensure that products are safe to use. Could something like this become the norm in AI?