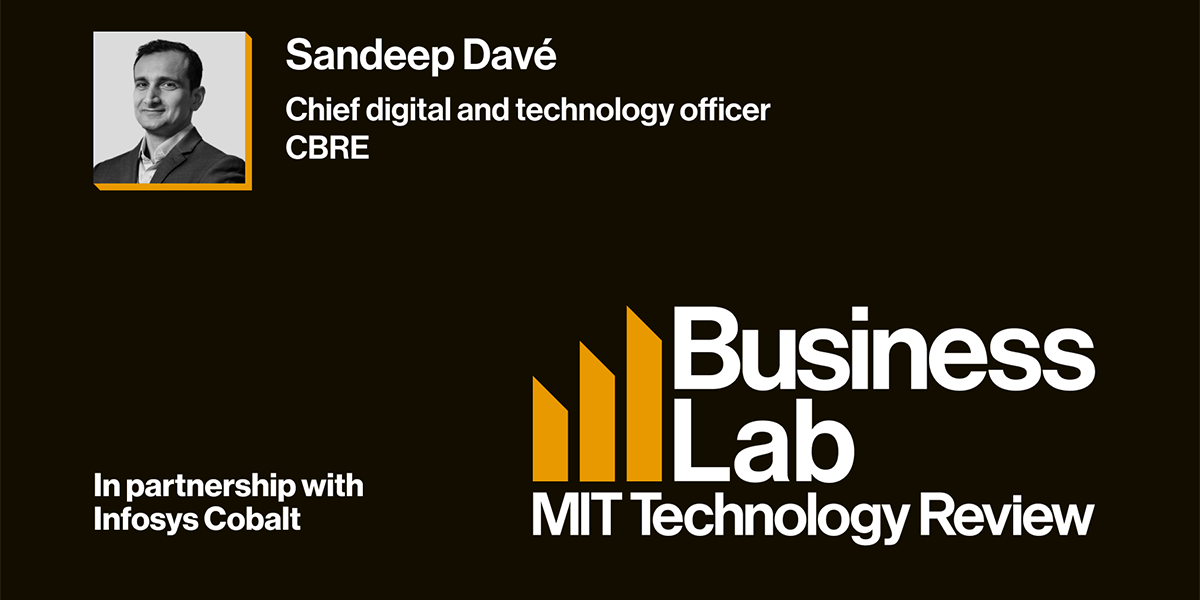
Sandeep: Sure. Using an example is great because this is such a wide field, both commercial real estate and the application of AI/ML in commercial real estate. In the area of smart buildings, we are focused on enabling three outcomes for our clients: energy, efficiency, and experience; which is how do they manage their energy usage, how do they get more efficient in everything that they do with respect to managing a property? And then what is the workplace experience for the employees in a building?
And let me just take an example of efficiency. There was a certain way in which buildings were managed previously. And with the application of cloud native global technology solutions, that we have that are infused with AI/ML, we are now able to manage facilities in a smarter manner, what we call Smart FM. We are able to look at occupancy and dynamically clean the environment rather than having people cleaning the environment on a regular schedule, we are able to save our clients a lot of money with respect to dynamic cleaning. We are able to detect anomalies in how we manage buildings and assets, which can then further reduce the false alarms and the number of truck rolls that need to happen with respect to managing a building. So there are so many different ways in which we infuse AI/ML.
Laurel: That’s really interesting. So according to a 2019 International Energy Agency global status report, the real estate industry contributed 39% of global carbon emissions. Could you offer us an example of how smart technologies, like what you’re talking about now, could boost operational efficiencies and then also help reduce emissions and improve sustainability?
Sandeep: Yeah, absolutely. I think there are two ways in which we look at this space. As you indicated that 39% of carbon emissions are contributed by real estate, and so therefore the industry has a huge role to play. Part of those emissions are at the time of construction itself, and the remainder is for the life cycle of the asset. Right at the time of construction, we’ve built capabilities where we are able to design and redesign based on a certain energy emission target for a building. We are able to select our suppliers based on a certain energy emission target for the building.
And then at the time of managing the building, there are many solutions that offer instant gratification, stick sensors up, light up a building, and they all work well if all you need to do is to light up a building. But in order to meet the scale and the global net-zero targets that our clients have set, our solutions need to be at portfolio scale and need to be multidimensional.
And so therefore what we do is we have the ability to ingest data from various different sources, from sensors, and are able to harmonize that and land it against a standard taxonomy. And then we are able to assess that in many different ways. We are able to bring together different aspects of looking at energy and looking at occupancy and managing the building based on the occupancy in the building. Those interventions, for example, at one of our clients recently, meant we were able to stand up those interventions at 25-plus buildings. And that led to a reduction in peak usage energy for them and also reduction in reactive maintenance work orders, reducing truck rolls, and supporting their energy goals.
Laurel: So you also are talking about this on a portfolio level. And CBRE’s own corporate responsibility and environmental social and governance or ESG goals are as follows: scale to a low-carbon future, create opportunities for employees to thrive through diversity, equity, inclusion initiatives and to build trust through integrity. How is CBRE using emerging technologies like artificial intelligence and machine learning to then become more efficient and also meet those ESG goals?
Sandeep: I think a lot of the ESG problem is a data problem. Today, if you talk to most who are trying and most are grappling with this problem right now, what they’ll say is that do they have a clear line of sight of what their, for example, scope 1 and scope 2, scope 3 emissions are? Are they able to capture the data in a reliable manner, audit it in a reliable manner, and then report against it? While they report against it, can they also manage usage? Because if you are able to look at the data, then you will know where corrective actions are required. Building on the foundation of the data platform that we’ve built on, which is 100% cloud native, by the way, we can then, on top of that, apply these technologies where we can apply ML models to detect anomalies. We take a digital twins perspective to map our data against the buildings and manage the end-to-end lifecycle of that real estate process.